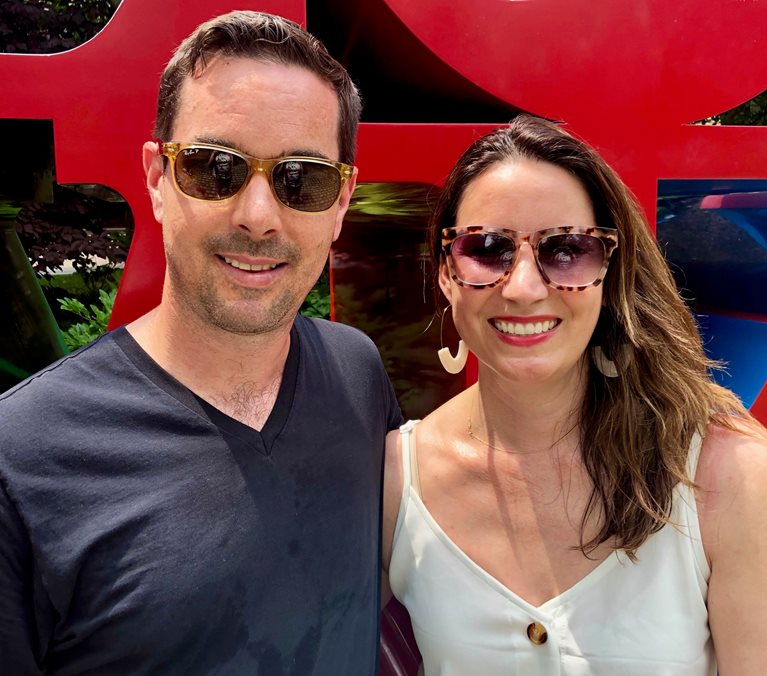
Before McKinsey, I worked for multiple start-ups, and honestly, wasn’t interested in consulting. I went to business school with people who had gone into the field, but I didn’t think it was for me. Still, when a recruiter contacted me two years ago about QuantumBlack, a McKinsey company, I said “yes” to learning more because it felt like a start-up environment within the firm. Through interviews, I discovered it was a good fit for me culturally and the work sounded exciting.
Upon joining, I was instantly blown away by the experience. Within the first month, I went to London for a week for training with the QuantumBlack team. I appreciated that the firm took the time to embed its culture in new hires. Then, I participated in a data engineering bootcamp in Connecticut. It was an intense 8:00 am to 7:00 pm classroom-type training, and I loved it. It was stimulating to learn so much and be around others who were also eager to grow, explore and try new things.
Right after that training, I joined a large transformation project in Montreal and quickly discovered what it means to be a data engineer at McKinsey. We spend significant time at the client’s site interviewing system owners and working with the team on problem solving. Our work is hands-on and we move fast.
Client connections are at the root of the work
To ramp up on a new engagement, we seek to understand the business; having access to data doesn’t mean we understand it fully. Clients know their businesses better than we do, so we listen first. That level of client-facing experience is not common for most data engineers, but at McKinsey, I get to spend time working with clients before the data analysis starts.
How we go about getting their data varies. Typically, we move the data into our secure platform, or we carve out a niche within their existing resources in a cloud environment. We take slices of their operational data and build a pipeline that cleanses, aggregates, and tees up raw information for modeling. Then, we run algorithms on it to train and test the model and produce results. We conduct exploratory data analysis to find anomalies in the data or coverage gaps which could impact our ability to model a specific business process.
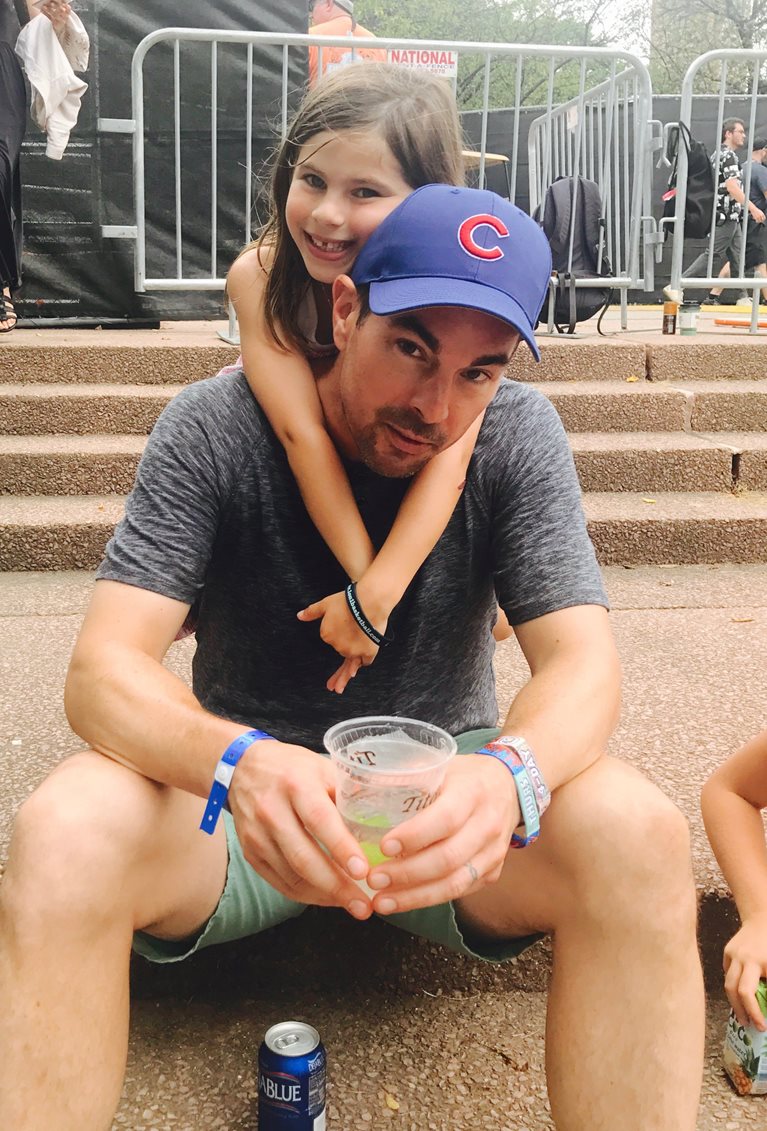
Meaningful work early on for a new data engineer
One of my first big projects was with an airline’s cargo division. During long-haul flights on large jets, they fill the plane with cargo after passengers board. However, weight restrictions apply, and our client needed to forecast how many passengers would board and account for luggage before selling cargo space. Adding to the challenge, for most destinations, there are multiple cargo options, and some airlines undercut others, driving a high volume of no-shows.
Our goal was to use advanced analytics and artificial intelligence to help our client book cargo more efficiently. What was cool about this project was we were forecasting how much space would be available and advising the airline how much to book based on expected no-shows, which was more than the forecast said would be available.
We worked with executives in the cargo group and embedded our model results into the tool they used daily. Before I joined, the team on the ground had already built the cloud platform. I got them to move to Azure and systematically publish all their operational data tables to a data lake. This level of maturity enabled us to jump into their data lake and deploy the software using their new architecture.
My first four months were spent getting out a Minimum Viable Product. Then we cherry-picked a set of meaningful routes and built the software and our model against them. After we started delivering value, the next phase of the project was bringing in a tech delivery lead and machine learning engineers to help scale the model, essentially taking what we built in the “lab” and moving it to the “factory.”
As a result, our clients went from having planes 60% full on average to 85% full. Creating change at the operational level separated this experience as a data engineer from many others. In this case, we built software that drove behavior changes.
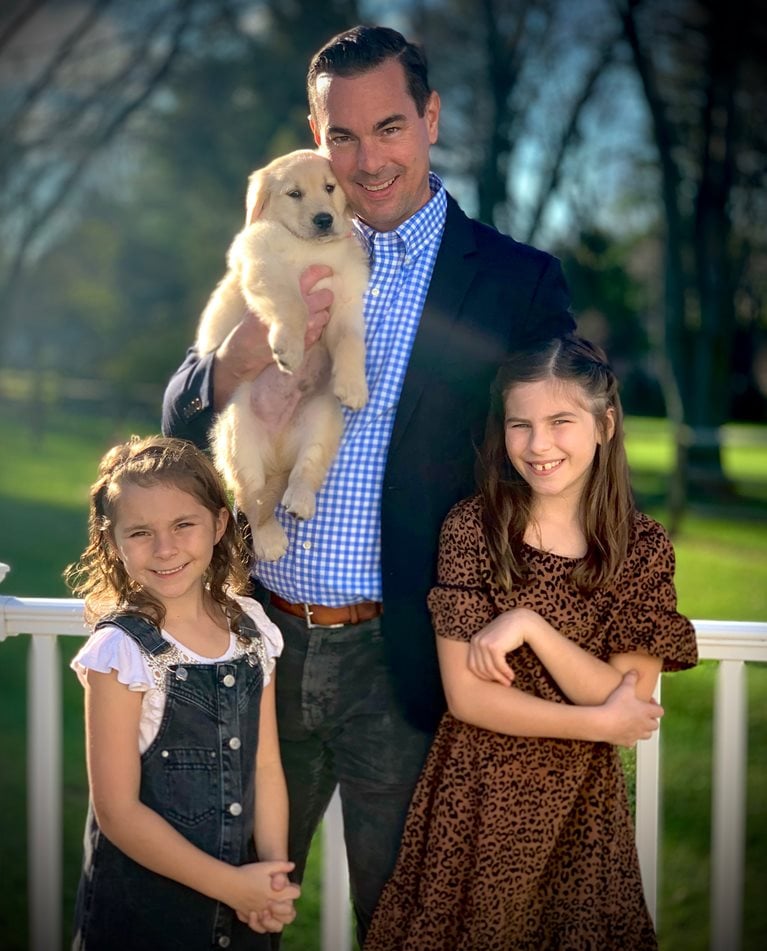
Finding job satisfaction in consulting
At McKinsey, I work in an academic culture with super smart people. I have a strong network of data engineers and data scientists who help me think about and apply the latest and greatest tools and techniques. Being in consulting requires you to jump into a brand-new environment and get things done on a timeline. We have to be creative and work quickly. I never get bored, and that’s why I love it here.
More about Brendan
When I’m not working with clients, I love to play tennis to stay healthy and active. I even joined a club in Montreal during the airline project to meet new people and learn French. I recently moved back to my hometown of Doylestown, Pennsylvania, where I’m plotting my next move.
For more information on McKinsey's data engineering career paths, visit mckinsey.com/TechCareers